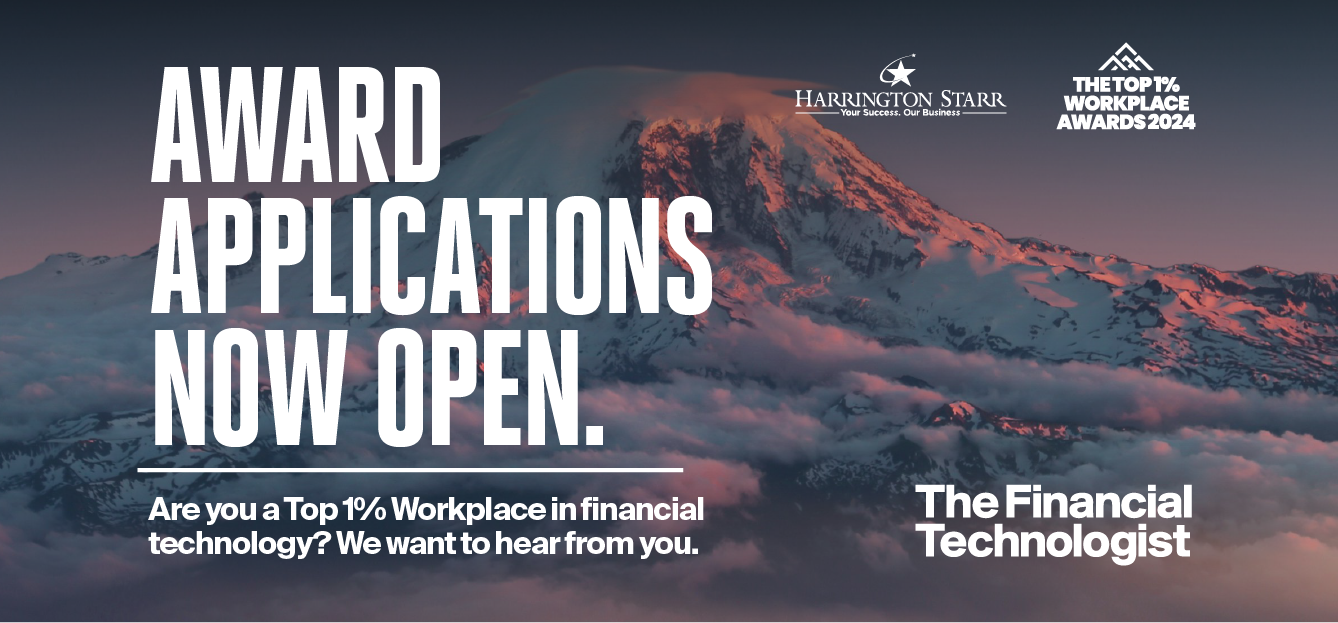
Download your free copy of the latest Financial Technologist magazine here.
The Asset-Based When asset- banks, investors, servicers, and Finance (ABF) industry has experienced significant growth since its inception in the 1980s. The market has evolved and expanded over time, encompassing various financial instruments such as asset- backed securities, mortgage- backed securities, collateralised debt obligations, and collateralised loan obligations. With a growing demand for alternative investments, technological advancements, ESG considerations, and an ever-evolving regulatory environment, the ABF market is set to continue its upward trajectory well into 2024.
Working in the structured finance industry can be challenging due to the complexity and non-standard nature of operational structures. To thrive in this field, one needs to constantly adjust to market changes by creating innovative financial and deal structures that are hard to standardise. The ABF industry, like many others, relies heavily on data processing. Due to the lack of standard frameworks, data formats, common reporting and monitoring procedures, workflow inconsistencies, errors, and inefficiencies in resource allocation and utilisation can occur. Furthermore, the value chain in this industry is very fragmented and poorly connected, and it has a wide variety of players, including sell-side (credit originators), servicing (credit structurers), and buy-side (institutional investors). Each player is highly dependent on the others and burdened by heavy and time-consuming reporting activities.
The need for advanced technology in the ABF market
In the current, highly-competitive landscape, speed and intelligence are crucial to secure deals. And yet, everyday players in the ABF market manage billions of credit investments using spreadsheets, manual workarounds, PDFs, and word documents. How can you make the right investment decisions and scale your organisation in the most efficient way possible? At Cardo AI, we believe the tools and technology used can have a significant impact on these goals. We commit to bridge the gap between people, data and technology in the ABF market by providing advanced data, software, and intelligence solutions to help value risk, optimise portfolios, and drive strategies. Our platform supports banks, investors, servicers and fund administrators to turn complex challenges into growth opportunities, starting from data management up to the most sophisticated predictive analytics, including cash flow projections. To date, we have supported over 220 million loan data on structured credit markets and ocwe 1,800 SPV data, while ensuring the highest standards in terms of accessibility, security, and scalability.
At Cardo AI, we apply artificial intelligence to help assess new opportunities without using extra capital or human resources:
■ Data analysis: AI can quickly and accurately analyse large volumes of data, enabling lenders to identify difficult-to-catch trends and patterns manually. With this information, lenders can make better decisions about which loans to approve and how to structure them.
■ Risk assessment: AI analysis of borrower data provides lenders with a more accurate creditworthiness assessment, improving loan decisions.
■ Product and services innovation: AI can help innovate ABF's product proposition. For example, it can create flexible pricing models that modify the interest rate of a loan based on the borrower's creditworthiness and the current market situation. Additionally, it can also be utilized to develop innovative forms of collateral that can be employed to secure a loan.
Machine Learning Models
Machine learning models play a crucial role in finance by estimating target outputs using predefined and structured data. These models can be trained to perform tasks like classification, regression, and forecasting. In the context of ABF, machine learning models help assess the profitability of consumer and SME loans by predicting factors such as default probability, time to default, prepayment probability, and ROI.
Quantitative Finance Models for Investment Optimization
Quantitative finance models, another facet of AI in finance, leverage advanced mathematical and statistical techniques to optimise investment allocation and price-targeted assets. These models enable AI to learn from data, identify patterns, make predictions, and make data-driven decisions. In ABF, quantitative finance models aid in cross-sector spillover analysis, market intelligence, and portfolio optimisation.
Language Models for Unstructured Data Analysis
Language models, a subset of AI, enable the analysis and interpretation of unstructured data such as text, images, and videos. Techniques like natural language processing (NLP), computer vision, and deep learning empower AI to understand human language, recognise images and videos, and make sense of complex information. While sounding unrelated initially, this technology can make a big difference in the realm of asset-backed securities and lending. Language models can automate loan document information extraction, generate insights from private debt datasets, and facilitate data mapping using natural language.
The adoption of AI in the ABF market is still in its early stages. However, it has the potential to revolutionise this sector. With more experimentation and positive outcomes, confidence in its use will continue to grow at a rapid pace. Cardo AI will keep exploring ways to enable banks and investors to act faster, manage better, and run cost-effective ABF transactions.
Download your free copy of the latest Financial Technologist magazine here.