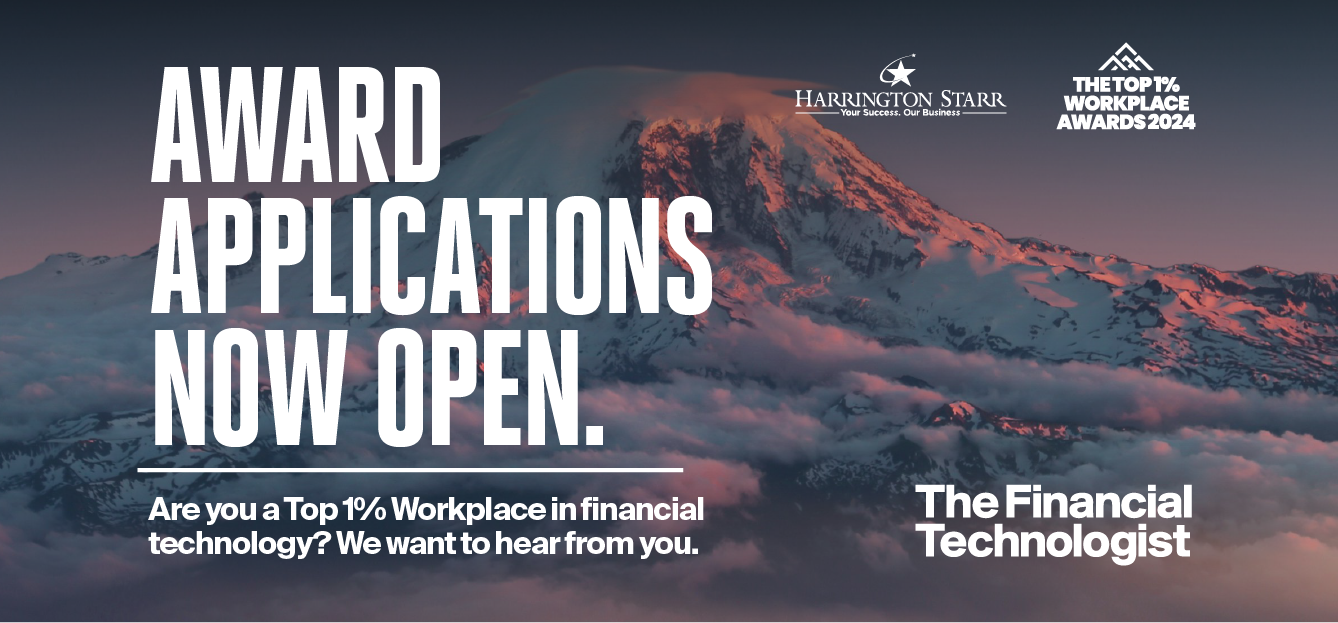
Download your free copy of the latest Financial Technologist magazine here.
We at ALFO are Leveraging organisations to tailor their risk thrilled to have been recognised as one of Harrington Starr’s 'Most Influential Financial Technology Firms of 2024.' Since our inception in April 2021, our guiding principle has been to change real-time, multi-asset analytics for risk, margin management, and pricing through disruptive innovation. In 2023, we achieved this vision by launching our modular product that encapsulates all these functionalities.
Key advantages of working with multiple risk models
In the complex domain of financial risk management, leveraging multiple risk models offers an effective strategy to tackle the multifaceted challenges posed by fluctuating markets, stringent regulatory requirements, and operational hurdles. This brief analysis explores the benefits of adopting a multi-model approach, underscores the importance of data quality, and examines the transformative impact of artificial intelligence (AI) on risk management practices.
At the heart of risk modelling is the foundational concept that risk equals the monetary value of potential loss multiplied by the probability of its occurrence. While models such as Value at Risk (VaR) have been pivotal, they exhibit limitations in scalability and in their ability to aggregate risk across diverse asset types. This limitation underscores the necessity of employing a broader spectrum of risk models, particularly for funds that navigate high-risk portfolios
and for multinational banks that operate across varied geopolitical contexts. The need for a flexible risk assessment approach is further emphasised by the unique risk profiles that different geographical regions may present, necessitating customised risk models to accurately evaluate geopolitical risks.
The regulatory environment adds another layer of complexity to risk management. Fund managers often face the challenge of adhering to different regulatory frameworks based on the jurisdiction of their funds. This scenario highlights the need for adaptable risk models capable of satisfying diverse regulatory requirements, illustrating how varying regulations, such as those from the European Securities and Markets Authority (ESMA) and the UK's Financial Conduct Authority (FCA), necessitate a dynamic approach to risk assessment.
Addressing these challenges, a modern risk management solution facilitates the integration of multiple risk models within a singular platform. This system allows for the concurrent application of various risk methodologies, enabling organisations to tailor their risk assessment practices to the unique needs of different operational levels. It effectively offers users the flexibility to select the most appropriate risk model for their specific circumstances, whether influenced by regulatory mandates, business model considerations, or strategic risk evaluation preferences.
Financial Risk Management is only as good as the quality of the data
The integrity of any risk management strategy is intrinsically linked to the quality of the data upon which it is based. The principle of "Garbage In, Garbage Out" is especially relevant, where subpar data quality can result in significant financial repercussions or compliance penalties. The importance of effective data management and optimisation of warehousing processes cannot be overstated, as these are crucial for ensuring accurate risk reporting and facilitating informed decision-making. This underscores the need for meticulous data verification to prevent the introduction of errors into the risk management process.
Artificial Intelligence and Risk Management
Artificial intelligence stands as a transformative force in risk management, enhancing the ability to analyse vast amounts of data for insights into potential risk factors. By employing AI technologies, organisations can transcend traditional analytical limitations, uncovering patterns and predictions that were previously unattainable. This advanced analytical capability enables a proactive approach to risk management, allowing organisations to anticipate and mitigate potential risks before they materialise.
One example of complex trend analysis or conditional probability: Movement in the price of Stock A caused movement in the value of Stock B and C. Market participants reacting to that may create movement in the price of Stock D and E to protect their positions in Stock F. Conditional probability can be used to assess whether movement in D and E were prompted by the movement in A and the reactions of B and C; or was a natural movement as result of fundamental economic factors? The overall aim is to support trading decisions in any or those stocks, for instance deciding whether to trade any of those shares, divest or simply wait for prices in those stocks to stabilise.
In conclusion, the journey towards integrated risk management is marked by the strategic use of multiple risk models, the leveraging of advanced technologies, and a meticulous focus on data quality. The incorporation of AI into this framework offers a forward- looking perspective, promising a new era of risk management characterised by predictive accuracy and strategic foresight. As the financial industry continues to navigate a landscape of uncertainty and opportunity, the adoption of these comprehensive risk management practices will be indispensable in achieving sustained success and resilience.
Download your free copy of the latest Financial Technologist magazine here.